Objectives of ZOOF A67
Brabant in-car III is a grant programme that falls within the framework of the national Better Use programme. It focuses on solving concrete traffic flow problems. Concrete results must be delivered by 2014 at the latest. Brabant In-car III focuses on using in-car technology to solve concrete traffic flow problems on the A67. In doing so, Brabant in-car III is the first of this series of programmes in which solving a concrete traffic problem was central.
The Brabant in-car III programme concerned a search for:
“ITS applications that optimise the interaction between cars and trucks in traffic so that the flow of both freight and passenger traffic improves. The scope of application includes at least the A67 (Belgian border to German border) and its entrances and exits. Measures must not compromise the network performance of the overall regional road network”.
Approach project
Brabant in-car III is the follow-up to the Brabant in-car I and II programmes. Unlike the previous two programmes, which housed a variety of projects, the current programme houses three projects with a high degree of similarity:
- Each of the projects focuses primarily on the development of a smartphone app on which in-car advice is given.
- The advice covers aspects such as driving speed, distance to the preceding vehicle and lane.
- These are given to drivers of passenger cars and trucks that regularly use the A67 between the Belgian and German borders on the route Antwerp via Eindhoven to the Ruhr region.
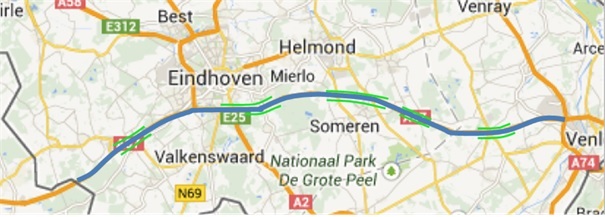
- Each of the projects uses knowledge developed in the HTAS project Connected Cruise Control (CCC).
- The TUD therefore plays a role in each of the projects.
- Project goals are improved traffic flow and road safety on the A67 now and roll-out of this service to other parts of the Netherlands and beyond as a follow-up goal.
V-tron participated in the subproject ZOOF (formerly Doorstroomrijden), which was carried out by Fourtress, Spr!ng, V-tron, Be-Mobile, TIM Digital Marketing, ProDrive and Fantasm.
Contact V-tron:
Results achieved
ZOOF made some improvements to the application and the back-end in the final phase of the project. As a result, two datasets were available for ZOOF. From the first dataset, the same overview features could be derived as in the Smoover and Blinkr subprojects.
Overall, it can be said that ZOOF as an app is taking over the function of the AID. Unfortunately, the timing of advisories and congestion detection is not quite perfect yet. This is therefore something that is very difficult to get done without being directly linked to roadside systems. The information chain preceding such an app usually contains too much delay to provide timely congestion warnings. A prediction algorithm or model can be used to compensate for that delay.
This is therefore one of the important differences from the November version of the app. In February, the app did work with a prediction model, before that it did not. The results above show that there is nevertheless still (more) to be gained in this. Timing and detection could be further improved.
Conclusions
For all three projects, it mainly seems that users drive with the flow of traffic. Therefore, you cannot expect that when the vast majority of traffic is not advised, app users will drive at significantly different speeds from the flow of traffic.
Whether users adjust their speed in response to the advisories, or whether the advisories match the traffic flow is hard to say. In that sense, it is a bit of a chicken-and-egg situation. To really properly analyse the behavioural effects, users’ speed profiles should be juxtaposed with the entire traffic flow. If clear differences then emerge shortly after advisory moments, there is a good chance that behavioural influence is taking place. However, such measurement was not feasible within this project.
Because users seem to largely drive with the traffic flow, it is also difficult to determine whether there are not subtle effects after all. If an advisory of 80, while driving 100, will people perhaps drive 95 km/h after all? This cannot be deduced from the speed profiles, and the averages also eliminate such effects. By diving deeper into the data, it might be possible to detect such effects, but even then it is questionable whether this effect is due to the advisory, or to the traffic flow.
As a kind of traffic jam-warning apps, Blikr! and ZOOF have potential. Blikr! in particular seems well capable of giving timely braking advice before reaching a traffic jam. With ZOOF, the timing and detection still needs some polishing.